Intro VMC - modeling and computing
Stat 221, Lecture 3
@snesterko
Lecture plan
- Intro.
- Modeling and computing material.
- Computing classification of models.
- Intro to computing with Odyssey.
- Thinking like a programmer - pseudocode, class languages.
- Critiquing a visualization, pset 1 information.
Announcements
- Final projects out.
- Ranking survey out soon.
- Piazza is good, use it.
Lecture 2 recap
- Distinctive features of parametric stat modeling.
- Ingredients of a stat model.
- Finding the estimand.
- Telling a story with the model.
- Agile movement setup \\( \longleftrightarrow \\) modeling.
- Parsimony in modeling.
- Visualization for model design.
Lecture 3 focus
- Problem setup.
- Method design.
- Implementation.
- Reporting.
Specifically, we will look at the connection and tradeoffs between modeling and computing.
Setting
- Survey done with RDS, need to estimate population mean.
- The researchers are using naive mean model which has a non-ignorable problem.
- We need to decide on a better method.
Used approaches
- Classify models in terms of computing intensity.
- Easily switch between workflow stages, and available options within a stage.
- Think a little like a programmer.
Why this is important
naive MCMC, vEM, HMM, GLM+HMC
Being able to navigate the tradeoffs in concrete applications is necessary to make the right choices.
Statistical models classified
Simple models:
- Regressions (logistic, linear), mixed effects regressions (somewhat).
- Autoregressive models.
Simple-to-complex models:
- Generalized linear models (GLM), spline/smooth regressions.
Complex models:
- Models with missing data/latent variables, HMMs.
- GLM's without the L, models from above combined.
Need new models and faster algorithms
- Standard models often don't work.
- Increase in the amount of available data - 2.8 trillion GB in 2012.
- Less and less cookie-cutter settings and challenges.
- Personal computers cannot handle large datasets, need distributed computing such as Odyssey.
Parallel computing
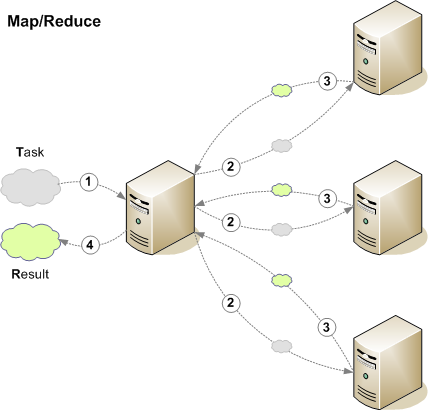
Decision sequence
- Design a model.
- Work on selecting a good computing technique for it, evaluate findings.
- Go back to either look for another computing technique, or change the model if the results are not satisfactory.
Example: naive model for RDS
- Simple model: $$Y_i \mathop{\sim}^{\rr{iid}} N\left( \mu, \sigma^2\right)$$
- Can be estimated on the personal computer easily.
- But CI coverage rates are bad. The model is not a good representation of the underlying mechanism.
- Go back to the design phase.
Recap: HMM
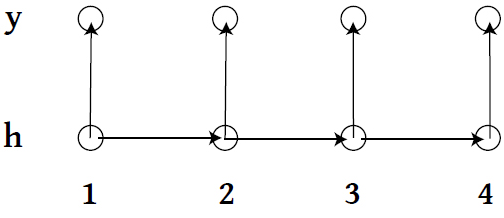
- \\(\{h_1, h_2, \ldots \} \\) live on discrete space, follow a Markov Process with a transition matrix \\( T_\theta \\).
- At time \\( t\\), state \\( h_t \\) emits observation \\( y_t \\) according to some specified model.
AP: first candidate
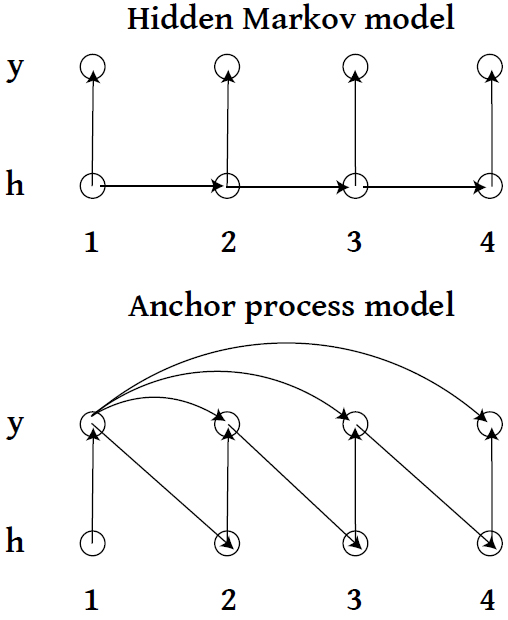
First candidate example

- Why would this be a bad candidate?
AP: second candidate
$$$$\begin{align} Y_i \given Y_{r(i)} & \sim N \left(\rho Y_{r(i)} + (1 - \rho) \mu, (1 - \rho^2) \sigma^2 \right), \\Y_i & \sim N\left( \mu, \sigma^2 \right) \rr{ if } r(i)\rr{ doesn't exist}\end{align}$$$$
- Needs Gibbs sampler to fit.
- Mostly analytic updates.
- Needs distributed computing for sensitivity analysis and coverage rate analysis.
- Provides good interpretation and interval coverage properties.
Benchmark: coverage
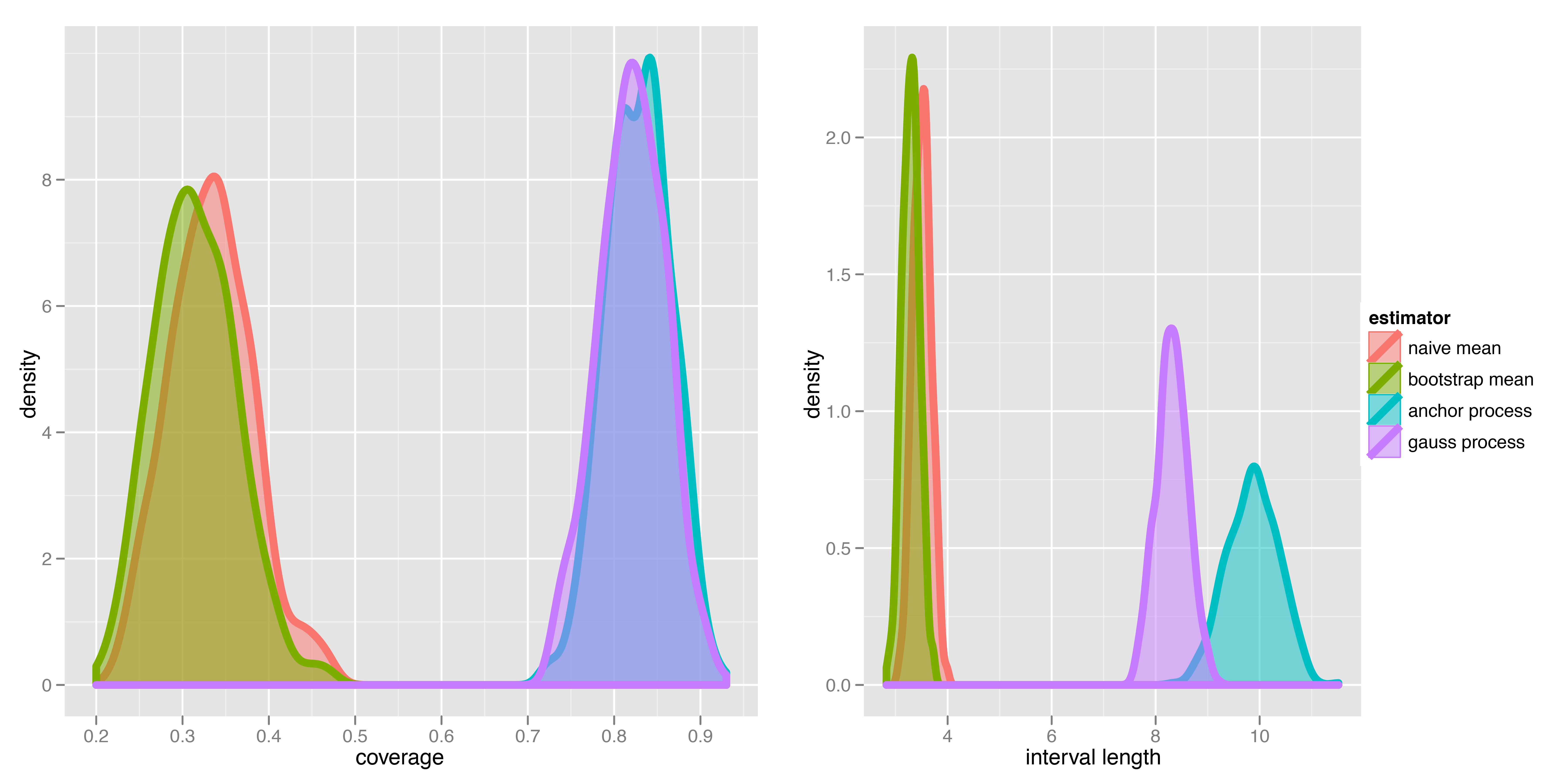
Winner
- Second candidate.
- Numerically stable (not so many parameters/missing data).
- Other favorable features.
What drives the decision?
- The estimand.
- Model performance metrics.
- What models are available/can be adapted?
- Work towards parsimony.
Choice of algorithm
- Numeric stability.
- Cost of implementation and execution.
- Point estimation vs. full sampling approaches.
- Point estimation: MLE (via LP, QP approximations, EM, Fisher scoring, simulated annealing), MAP.
- Full sampling: MCMC, HMC, Gibbs, exact sampling.
Thinking like a programmer
Pseudocode
Simulate from the simple model:
for i in 1:N
generate and store Y_i from Normal(mu, sigma-sq)
Simulate from the AP model
for i in 1:N
referrer = r(i)
if referrer
Yr = Y_referrer
generate and store Y_i from Normal(rho * Yr + (1 - rho) * mu, (1 - rho^2) * sigma-sq)
else
generate and store Y_i from Normal(mu, sigma-sq)
Pseudocode for main fitting function
initialize parameter values
for i in 1:ndraws
## this is Gibbs sampler
draw mu analytically given current rho and sigma-sq
draw rho via Metropolis proposals on transformed space given current rho and sigma-sq
draw sigma-sq analytically given current mu and rho
discard first nburnin draws of parameters
Pseudocode becomes R code
tr = init(data)
for (ii in 1:settings$ndraws) {
## this is Gibbs sampler
pars = lastParams(tr)
tr$mu = combine(tr$mu, drawMu(data, pars))
pars = lastParams(tr)
tr$rho = combine(tr$rho, drawRho(data, pars))
pars = lastParams(tr)
tr$ss = combine(tr$ss, drawSigmaS(data, pars))
}
tr = discard(tr, settings$burnin)
tr
Pseudocode becomes good R code
## initialize parameter values
tr = init(data, settings)
## perform the main Gibbs sampler loop
for (ii in 1:settings$ndraws) {
## lastParams gets the most recent parameter draws
pars = lastParams(tr)
tr$mu = combine(old = tr$mu, add = drawMu(data, pars))
pars = lastParams(tr)
tr$rho = combine(old = tr$rho, add = drawRho(data, pars))
pars = lastParams(tr)
tr$ss = combine(old = tr$ss, add = drawSigmaS(data, pars))
}
## Burn in - throw away first burnin draws set in settings
tr = discard(allparameters = tr, nthrow = settings$burnin)
tr
Good code
- Saves time.
- Easier to handle new languages.
Class computing languages
R
for (ii in 1:10) {
print("R is awesome")
}
Bash
for ii in $(seq 1 10)
do
echo "R is awesome"
done
- Many code snippets provided in homeworks.
Class visualization languages
Javascript (optional), snippets provided
for (var ii = 0; ii < 10; ii++) {
console.log("R is awesome");
}
CSS (optional), snippets provided
svg line {
stroke : #000000;
opacity : 0.3;
}
HTML (optional), snippets provided
<html>
<body><p>R is awesome</p></body>
</html>
Why need fast code in R
- Personal to distributed computing leap.
- short_serial, short_parallel queues on Odyssey.
- Computing resources are usually constrained.
How to write fast R code
- Vectorization.
- Think in terms of matrix operations.
- Vector indexing.
- Built-in functions and functions in packages, some are in lower level languages and so are faster.
- (Hint for pset1: may want to consider xpnd in MCMCpack).
Example of fast code in R
time1 <- proc.time()
res1 <- sapply(1:1000000, exp)
time2 <- proc.time()
time2 <- time1
## user system elapsed
## 6.321 0.146 6.429
time1 - proc.time()
res2 <- exp(1:1000000)
time2 <- proc.time()
time2 <- time1
## user system elapsed
## 0.022 0.016 0.051
all(res1 == res2)
## [1] TRUE
Problem set 1 visualization
Announcements
- Submit your final project rankings by February 10 at noon!
- Odyssey access: send your name, email, and phone # to your TF.
Final slide
- Next lecture: guest lecture by Rachel Schutt